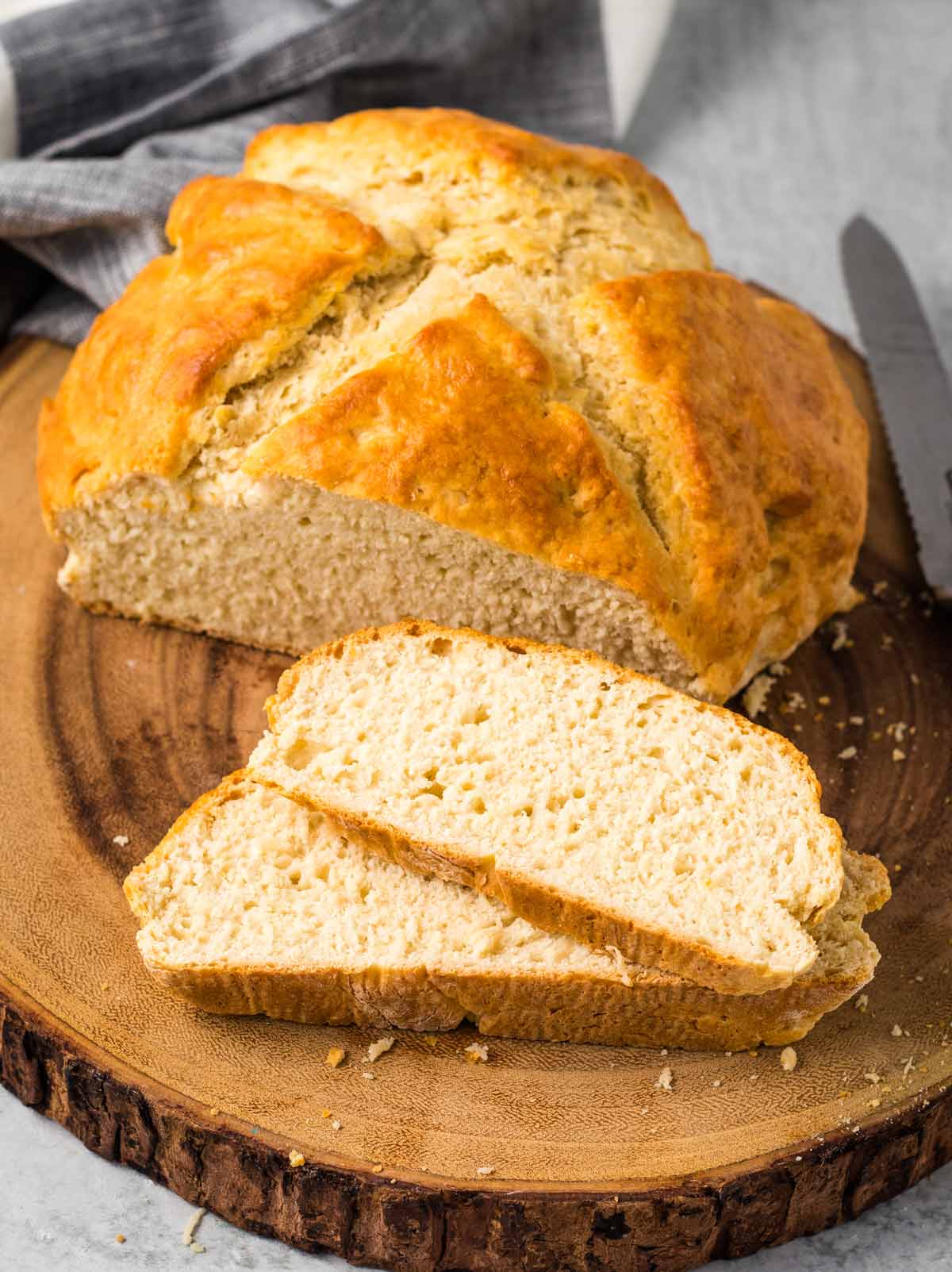
Understanding P-Values in Modern Statistics
In the realm of statistical analysis, the p-value plays a crucial role in hypothesis testing. It provides a measure for determining the significance of results in various experiments and research studies. By understanding how to find the p-value, researchers can make informed decisions based on their data. This article will delve into the effective ways to calculate the p-value, interpret its significance, and explore its implications in different statistical contexts such as t-tests, ANOVA, and regression analysis.
Moreover, we will discuss the importance of the significance level in relation to p-values, providing real examples and applications. By the end of this article, you’ll have a comprehensive understanding of p-value calculation and its practical applications in hypothesis testing.
So, what are the key takeaways? You will learn about the p-value definition, how it influences decision making, and the threshold for considering results statistically significant.
Defining the P-Value and Its Importance
The p-value, short for probability value, is a statistical metric that helps quantify the strength of evidence against the null hypothesis. In simple terms, it indicates the probability of obtaining an observed effect or more extreme results under the assumption that the null hypothesis is true. Understanding p-value meaning is crucial for researchers as it directly influences their interpretations and conclusions.
The significance level, often denoted as alpha (α), serves as a benchmark against which the p-value is compared. Common practice sets this alpha at 0.05, suggesting that a p-value less than this threshold indicates statistical significance. Thus, knowing how to determine significance with p-value is key for hypothesis testing.
Furthermore, the interpretation of p-values must consider their context. For instance, a low p-value (<0.05) implies that the null hypothesis can be rejected, indicating that the alternative hypothesis holds more weight. Conversely, high p-value meanings suggest insufficient evidence to reject the null hypothesis.
Calculating the P-Value: Methods and Techniques
There are various methods available for calculating p-values, depending on the statistical test in use. Below, we review several effective techniques.
Using P-Value Calculators
Utilizing a p-value calculator is one of the most straightforward methods. These tools allow users to input their data and desired test type, providing instant results for the p-value. Many online platforms can perform calculations based on various tests, including t-tests, ANOVA, and chi-square tests.
Calculating P-Value by Hand
For more hands-on insight, calculating the p-value by hand can be educational. In a t-test, for example, after determining the t-statistic, you would compare it to the critical value from t-distribution tables. The area under the curve to the right of the t-statistic gives the p-value.
P-Value in Common Statistical Tests
Different statistical tests use specific methods to find the p-value. For instance, in ANOVA, the p-value helps determine whether there are statistically significant differences among group means. Understanding p-value in ANOVA not only aids in analysis but is also vital when discussing research findings.
As you explore deeper into hypothesis testing concepts, it becomes clear that the p-value plays a pivotal role across a multitude of tests.
Interpreting P-Values: From Statistics to Real-World Applications
Interpreting p-values correctly is crucial for making informed conclusions in research. A low p-value typically indicates that the results are statistically significant, but care must be taken to avoid misinterpretations. For example, reporting a small p-value does not imply a large effect size. It merely signifies that the data is inconsistent with the null hypothesis.
Low P-Value Interpretation
A low p-value suggests powerful evidence against the null hypothesis, often leading to its rejection. Researchers should also consider the context and implications of these findings, such as the relationship between p-value and confidence interval.
High P-Value Meaning and Implications
In contrast, a high p-value indicates a lack of significant evidence to reject the null hypothesis. It’s important to communicate that a high p-value does not confirm the null hypothesis; instead, it suggests insufficient evidence regarding the alternative hypothesis. This distinction is key in p-value hypothesis testing.
Common Mistakes with P-Value Interpretation
Misunderstandings about p-values can lead to significant errors in research. Common mistakes include treating p-values as definitive proof or failing to account for study design and sample size considerations. It's essential to comprehensively educate on the correct interpretation of p-values to prevent these pitfalls.
Critical Analysis of P-Values in Research
As with any statistical tool, p-values hold limitations that researchers must acknowledge. The reliance upon a fixed threshold for p-values can overly simplify complex results. In recent years, the statistical community has debated the inclusion of p-values, with calls for a more holistic approach to data analysis techniques.
P-Value and Type I/II Errors
Understanding the relationship between p-values and type I and II errors is vital. A type I error occurs when rejecting a true null hypothesis, while a type II error reflects a failure to reject a false null hypothesis. The p-value threshold largely influences these risks, and researchers must be vigilant in their interpretation and reporting.
Reporting P-Value Results
Clear communication of p-value results is essential in research papers. Researchers should include not only the p-value but also the specific statistical test used, the sample size, and context around findings. This comprehensive reporting will enable readers to better understand the implications of the statistical results.
Conclusion: Embracing the P-Value in Data Analysis
The p-value remains a cornerstone of statistical analysis and hypothesis testing. A thorough grasp of its calculation, interpretation, and implications is critical for researchers across disciplines. By using p-values wisely, one can enhance data storytelling and make informed decisions that drive findings in modern research.
As we continue to navigate the evolving landscape of statistics, embracing a nuanced understanding of p-values will empower researchers to leverage them effectively in their work.
In closing, the journey to mastering p-values is still ongoing. With the right tools and a critical approach, researchers can unlock the full potential of statistical analysis and contribute meaningful insights to the scientific community.