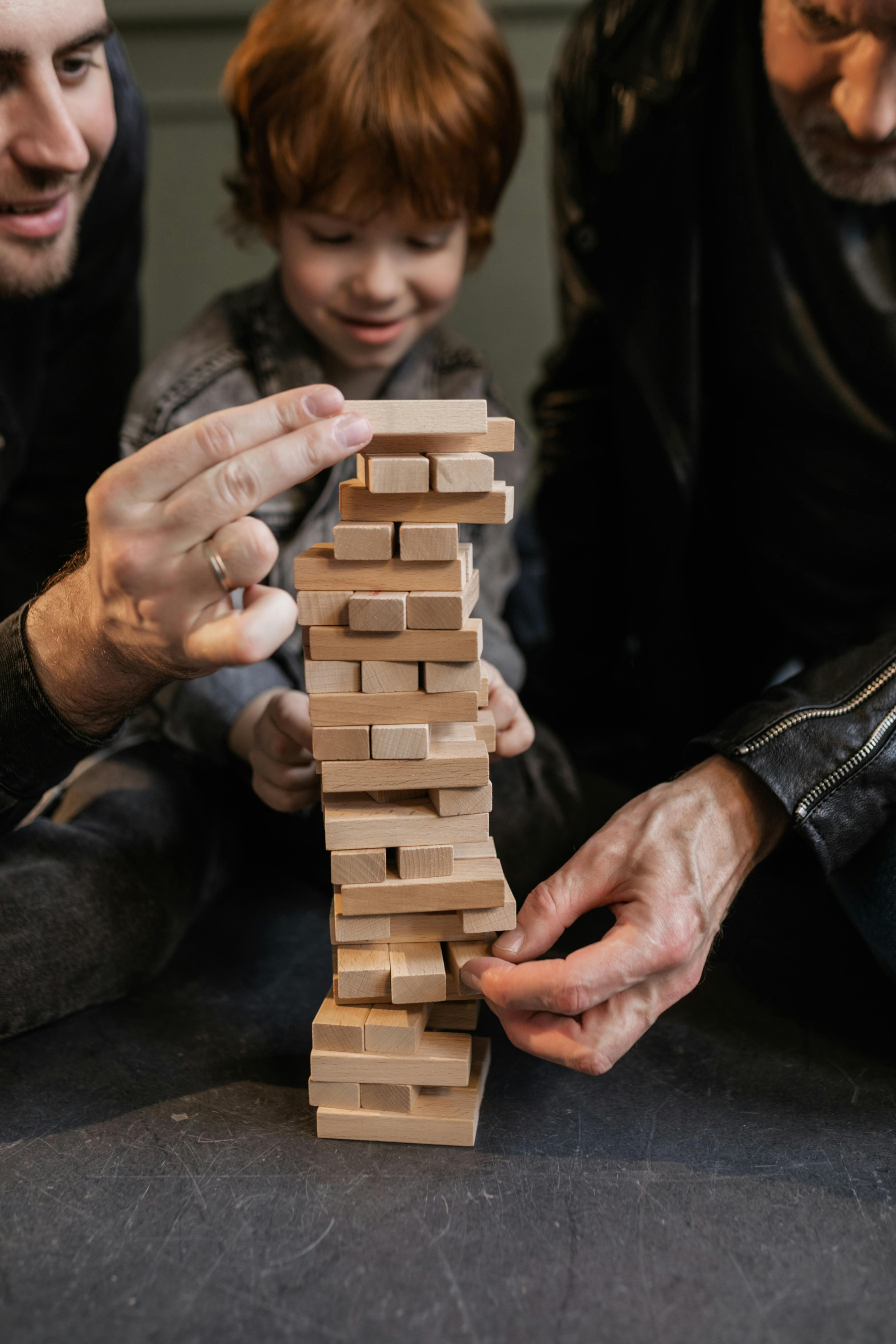
How to Properly Find the IQR for Better Data Analysis in 2025
Understanding IQR and its Importance in Data Analysis
The **interquartile range (IQR)** is a crucial statistical measure that helps analysts understand the spread and variability of data. By focusing on the middle 50% of a dataset, IQR provides insights into data distribution and is instrumental in hinting at the presence of outliers. The ability to assess data spread is especially relevant in data analysis tools, making the IQR a robust metric in many fields including finance, healthcare, and scientific research. Knowing how to calculate the IQR properly is essential for accurately interpreting results, allowing statisticians to identify trends or anomalies effectively. Thus, mastering the art of finding IQR for data is a valuable skill in 2025.
IQR Formula and Calculating Quartiles
The **IQR formula** is straightforward: it is calculated by subtracting the first quartile (Q1) from the third quartile (Q3). This can be expressed mathematically as: IQR = Q3 - Q1. To find Q1 and Q3, it's essential to organize the dataset in ascending order. Once sorted, the first quartile can be identified as the median of the lower half of the data, while the third quartile is the median of the upper half. This quartile calculation provides not only the middle 50% of the data but also informs us about the median, reinforcing how **IQR relates to median** in interpreting datasets effectively. Understanding these calculations sets the foundation for robust statistical measurements.
Why Use IQR in Descriptive Statistics?
The importance of IQR in descriptive statistics cannot be overstated. It serves as a measure of statistical variability, essential for understanding the distribution of data rather than simply relying on the dataset's range. IQR is robust against outliers, which means it accurately reflects the spread of the data without distortion from extreme values. This characteristic makes it highly effective in applications involving IQR in box plot analysis. In graphics, box plots visually communicate the IQR, quartile values, and potential outliers clearly, which aids in data interpretation and enhances reporting standards.
Step-by-Step IQR Calculation and Identification of Outliers
Calculating the IQR accurately involves a few systematic steps that ensure clarity in data analysis. Following these IQR calculation steps allows for a more comprehensive understanding of a dataset. It’s also vital for organizations analyzing different dimensions of performance metrics from their data.
Steps for Finding the IQR
1. **Organize Your Data**: Begin by sorting your data in ascending order. This step is crucial for correct quartile calculation.
2. **Calculate Q1 and Q3**: Identify Q1 as the median of the first half of the sorted data, and Q3 as the median of the second half.
3. **Use the IQR Formula**: Subtract Q1 from Q3 (IQR = Q3 - Q1) to find your interquartile range.
Once you have calculated the IQR, determine the outliers using the lower quartile (Q1) and upper quartile (Q3) along with a multiplier (commonly 1.5). Any data point beyond Q1 - (1.5 * IQR) or Q3 + (1.5 * IQR) is regarded as an outlier. This method is essential in **identifying outliers with IQR**, making it valuable in robust statistical analysis.
Example of IQR Calculation in a Dataset
To illustrate the **IQR method**, consider a small dataset: [12, 15, 14, 18, 20, 21, 23, 36, 45]. Begin by sorting the numbers: [12, 14, 15, 18, 20, 21, 23, 36, 45]. Here, the median (Q2) is 20, with the dataset split into two halves: [12, 14, 15, 18] and [21, 23, 36, 45]. The first quartile, Q1, is the median of the first half: (14 + 15)/2 = 14.5. For Q3, the median of the second half is (23 + 21)/2 = 22. The IQR then is 22 - 14.5 = **7.5**. This example encapsulates how easy it is to quantify data variability using IQR, reinforcing its invaluable role in data quartile analysis.
Applications and Implications of IQR in Data Analysis
The utilization of IQR extends into various domains, making it significant for comprehensive data analysis and research methodologies. By understanding how to gauge variability and assess data distribution with IQR, businesses can refine their strategies and forecasting methods.
IQR in Box Plot Analysis
IQR is a primary component in constructing box plots, which provide an immediate visual representation of a dataset's distribution. The box in a box plot signifies the middle 50% of the data, with lines (whiskers) extending towards the minimum and maximum values (excluding outliers). This graphical representation simplifies **IQR measurement in datasets** and facilitates quick assessments of skewness and outlier presence, thereby saving time during data analysis and interpretation.
Robust Statistical Measurements Beyond IQR
Beyond merely calculating the IQR, it's beneficial to understand its implications in broader statistics. The ability to utilize the IQR as a threshold aids in decision-making, allowing teams to outline the importance of **IQR in descriptive statistics** and in constructing visual data analyses. Recognizing where datasets diverge from normative data patterns enhances inferential capabilities in statistical modeling and forecasting.
Key Takeaways and Conclusion
The **interquartile range (IQR)** is an essential tool for data analysts in 2025 who are tasked with discerning data distribution and variability. Its focus on the spread of the middle 50% of values allows for effective outlier detection and insights into dataset integrity. Mastery of the steps involved in finding IQR not only empowers the analyst with data understanding but also aligns with good practices in statistical reporting. By continually leveraging these concepts, issues in analytical techniques can be minimized and the potential for robust data interpretations maximized.
FAQ
1. What is the IQR formula?
The **IQR formula** is defined as IQR = Q3 - Q1, where Q1 is the first quartile and Q3 is the third quartile. This calculation helps determine the spread of the middle 50% of the data, making it a significant measure in understanding data variability.
2. Why is IQR preferred over range in statistical analysis?
The IQR is often preferred over range because it is less influenced by outliers. While the range considers all values in the dataset, including extreme ones, the IQR focuses solely on the middle data, making it a more **robust statistical measure** for analyzing variability.
3. How can I calculate the IQR in Excel?
In Excel, calculating the IQR can be done using built-in functions. You can find Q1 using PERCENTILE.EXC(array, 0.25) and Q3 using PERCENTILE.EXC(array, 0.75). The IQR can then be calculated by subtracting the two results. This straightforward method facilitates data analysis tasks efficiently.
4. How does IQR help in identifying outliers?
IQR helps in **identifying outliers** by calculating boundaries for acceptable data values. A common method uses 1.5 times the IQR to establish thresholds beyond which points are considered outliers, guiding analysts in effectively managing exceptional data points.
5. Can you give an example of using IQR in performance metrics?
Yes! In measuring employee performance metrics, IQR can help identify workers who perform exceptionally high or low. By applying IQR to performance data, organizations can discern which metrics fall outside expected performance levels, enabling focused improvements in workplace practices and individual training programs.
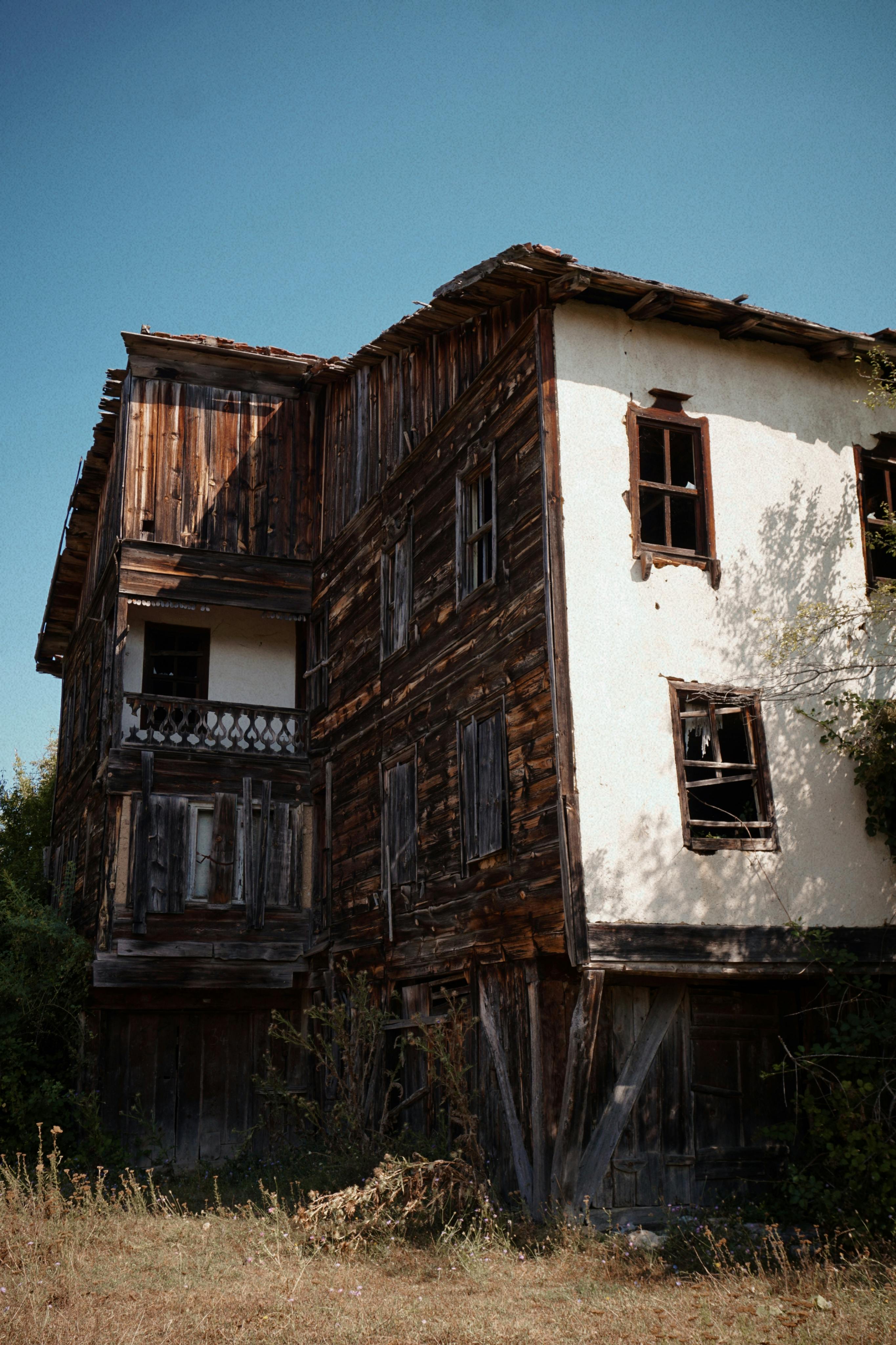
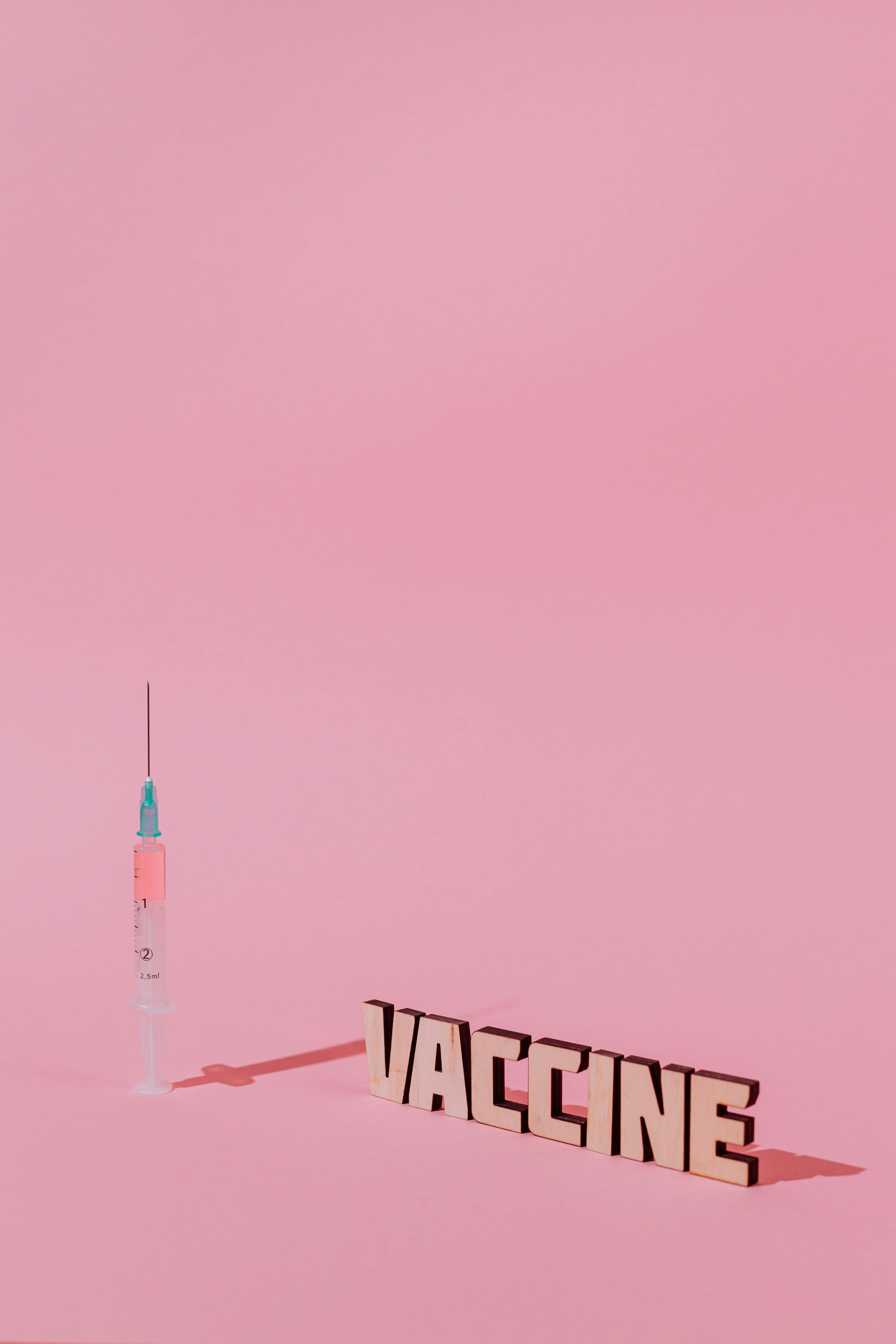