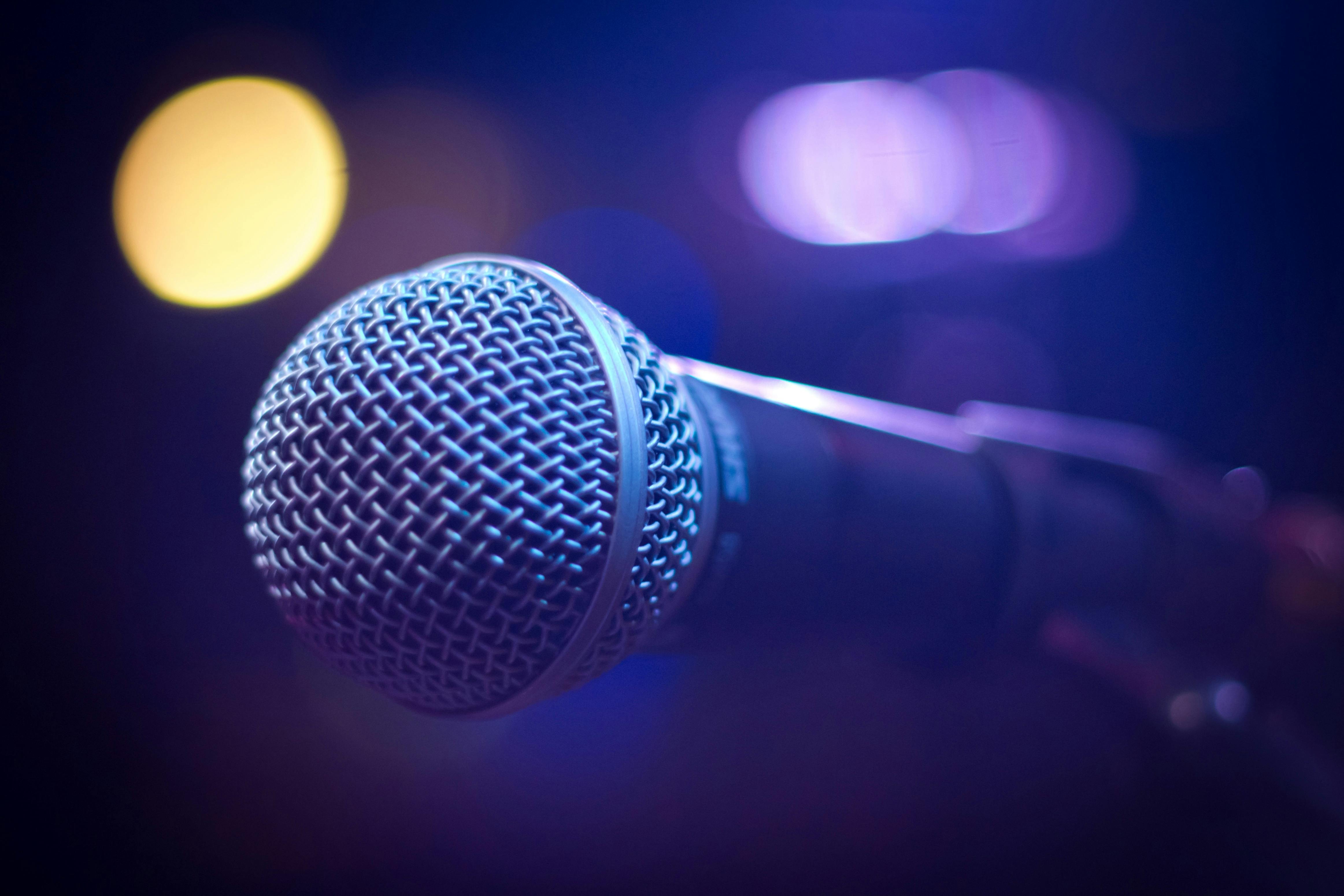
Smart Ways to Find Confidence Interval for Better Statistical Analysis in 2025
The Importance of Confidence Interval in Statistical Analysis
Understanding the **confidence interval** is crucial for effective **statistical analysis**. This range gives insights into the **population mean**, indicating where the true parameter lies based on the analysis of **sample data**. A **confidence interval** not only offers an estimate but also quantifies the **reliability** of this estimate, helping researchers make informed decisions. As we venture into 2025, harnessing robust techniques to calculate this interval becomes essential, especially when analyzing large datasets. By recognizing the role of key elements such as **margin of error** and **standard deviation**, analysts can better interpret their results and validate assumptions.
Understanding Margin of Error
The **margin of error** is a pivotal component in the calculation of a **confidence interval**. It represents the range of values that your sample estimates are expected to differ from the actual population value. For example, a **margin of error** of ±3% means if your sample mean is reported at 60%, you can reasonably expect the **population mean** to be between 57% and 63%. This understanding is fundamental in achieving a proper **interval estimation**. Analysts often use a higher level of significance, or a lower error rate, to ensure their **interval** is tight enough to reflect a true representation of data.
Statistical Significance and Confidence Levels
Another essential factor in finding a **confidence interval** is the choice of **significance level**. Typically, researchers use a 95% or 99% confidence level, which indicates how confident they are that the true population parameter lies within the calculated interval. A higher **confidence level** increases the **interval's** width, thereby increasing its reliability but also its uncertainty measurement. The balance between a high **confidence level** and a practically useful **confidence interval** requires a nuanced understanding of both **sample size** and **population variability**.
Practical Steps to Calculate a Confidence Interval
To effectively calculate a **confidence interval**, the following steps can be followed:
- Identify Key Parameters: First, gather your sample information including the mean, standard deviation, and sample size.
- Select a Confidence Level: Decide on the significance level (commonly 0.05 or 0.01) which influences the critical value.
- Calculate the Margin of Error: Using your selected critical value (z-score for large samples or t-distribution for smaller samples), you can calculate your **margin of error** using the formula: Margin of Error = Critical Value × (Standard Deviation / √n).
- Construct the Confidence Interval: Finally, create your **confidence interval** using: Confidence Interval = Sample Mean ± Margin of Error.
This systematic approach ensures accuracy in the **interval estimation** process.
Exploring the Role of Sampling and Data Characteristics
The quality of the **confidence interval** is highly dependent on the characteristics of the **sample** from which data is drawn. **Sampling distribution** theory, as encapsulated in the Central Limit Theorem, tells us that regardless of the **data distributions**, the means of sufficiently large samples will tend to follow a normal distribution. This assumes that **sample size** is large enough for the laws of statistics to operate effectively.
Impact of Sample Size on Confidence Interval
Sample size plays a critical role in determining the width of a **confidence interval**. Larger samples tend to produce smaller **margins of error**, leading to narrower **confidence intervals**. For instance, if five respondents report a mean whereas a survey of 500 provides similar results, the latter will yield a much narrower **confidence interval** due to the enhanced ability to minimize **sampling error**. Thus, understanding how **sample size** impacts data variability is vital for precise parameter estimation.
Addressing Data Variability and Distribution
Understanding the **data variability** in your samples aids in calculating a valid **confidence interval**. For instance, a data set demonstrating high variability will necessitate a broader **confidence interval** to account for unpredictability. Employing tools such as histograms helps visualize such variability. Moreover, ascertain whether your data conforms to the **normal distribution** expectation or if adjustments are necessary using transformations to reduce skewness.
Utilization of Advanced Techniques: Bootstrapping and Bayesian Methods
For extracting more insights from data, particularly when dealing with complex data, bootstrapping techniques prove advantageous. This involves resampling your data many times, allowing you to estimate the distribution of a statistic (like the mean) and consequently construct a more robust **confidence interval**. Similarly, **Bayesian statistics** offers an alternative approach where prior knowledge and current data collaboratively inform your **interval estimation**, leading to better **interpretation** of findings compared to traditional methods.
Common Applications and Interpretation of Confidence Intervals
**Confidence intervals** have a broad range of applications across various fields of research and analysis, including **quantitative research**, survey analysis, and even experimental settings. The interpretation of a **confidence interval** goes beyond merely identifying the range; it's about communicating uncertainty, risk, and reliability to stakeholders. Properly interpreted **confidence intervals** can offer profound insights into policies, marketing strategies, and public health outcomes.
Utilizing Confidence Intervals in Regression Analysis
In **regression analysis** specifically, the interpretation of **confidence intervals**, such as the **proportion confidence interval**, helps to understand the predictive accuracy of models. They reflect the uncertainty around estimated coefficients, thus granting stakeholders a clearer view of the reliability in predictions made based on data-driven models.
Confidence Intervals in Hypothesis Testing
While conducting hypothesis testing, one often utilizes **confidence intervals** to assess whether sample estimates are consistent with what is hypothesized regarding the population. If a hypothesized value lies outside the calculated **confidence bounds**, then it can be considered statistically significant, leading to hypothesis rejection. Thus, understanding this relationship is crucial for effective data insights.
Key Takeaways
- Understanding how to calculate and interpret the **confidence interval** is vital for accurate statistical analysis.
- The **sample size** and **data variability** significantly influence the reliability of your **confidence interval**.
- Advanced methods like **bootstrapping** and **Bayesian statistics** improve estimation, providing more robust analytical frameworks.
- Clear communication of **confidence intervals** allows for informed decision-making in research and policy.
FAQ
1. What factors influence the width of a confidence interval?
The **width of a confidence interval** is primarily influenced by the **sample size**, **standard deviation**, and the chosen **confidence level**. A larger **sample size** leads to a narrower interval, while a higher **confidence level** broadens it.
2. How does the normal distribution affect confidence intervals?
The assumption of **normal distribution** allows for the application of standard statistical methods for calculating **confidence intervals**. With larger sample sizes, the Central Limit Theorem assures us that sample means will approximate a normal distribution, thus facilitating the interval calculation.
3. What is the difference between a prediction interval and a confidence interval?
A **prediction interval** predicts the range for a single new observation, while a **confidence interval** estimates the range of the population parameter. Hence, the **prediction interval** is usually wider due to the added uncertainty related to individual observations.
4. Can a confidence interval be used for qualitative data?
Typically, **confidence intervals** are designed for continuous quantitative data. However, proportion confidence intervals can be utilized for categorical responses to convey uncertainty regarding the proportion of each category.
5. How can bootstrapping enhance the calculation of confidence intervals?
**Bootstrapping** allows for the resampling of the dataset to build a distribution of the statistic, leading to more precise estimates. This approach can be particularly beneficial when conventional methods produce unreliable estimates due to sample limitations.